CX analytics is important for businesses to understand their customers to provide a better experience and improve customer loyalty. Predictive analytics uses historical data and machine learning algorithms to identify patterns and predict future outcomes, while prescriptive analytics uses those predictions to provide actionable recommendations.
Combining these two types of analytics can give businesses a powerful tool to predict customer behavior and prescribe actions to improve the customer experience and ultimately drive business outcomes. This combination can lead to better decision-making and a more personalized customer experience.
How Predictive and Prescriptive Analytics Fusion Works
Integrating predictive analytics and prescriptive analytics helps organizations gain a holistic understanding of their customers, enabling them to create targeted marketing campaigns, enhance product design, and improve customer service.
Predictive analytics predicts future outcomes using statistical and machine learning algorithms, while prescriptive analytics provides recommendations on optimizing business operations and customer experiences.
-
Predictive analytics informs prescriptive analytics by identifying customer behavior and preferences, allowing personalized experiences.
-
Prescriptive analytics, in turn, optimizes predictive analytics by recommending actions based on insights gained from data, such as specific retention strategies for customers at risk of churn.
-
Prescriptive analytics also improves data collection and analysis for predictive analytics, increasing the accuracy of predictions and helping organizations make better decisions.
-
The integration of prescriptive and predictive analytics can improve customer satisfaction and loyalty through targeted experiences.
6 Steps to Implement the Blend of Predictive and Prescriptive analytics
Implementing predictive and prescriptive analytics involves several key steps to ensure organizations can effectively leverage both analytics to drive business outcomes. Here is a
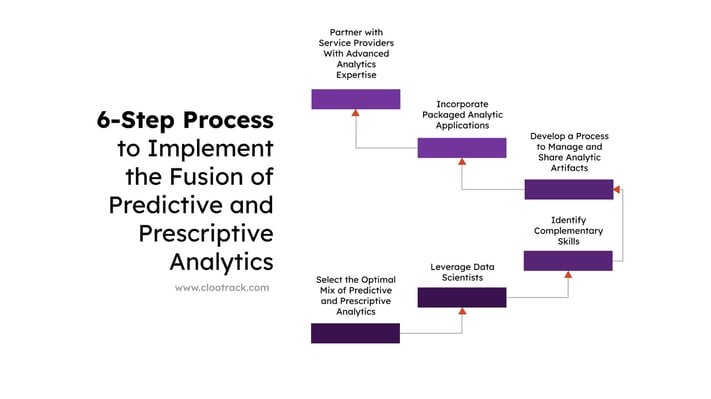
This section will elaborate on selecting the optimal mix of analytics capabilities, hiring or training data scientists, identifying complementary skills, developing a process to manage analytic artifacts, incorporating packaged analytic applications, and partnering with service providers with advanced analytics expertise.
Step 1: Select the Optimal Mix of Predictive and Prescriptive Analytics
The first step is to select the optimal mix of predictive and prescriptive analytics capabilities. Here are the steps to select the optimal mix of predictive and prescriptive analytics:
-
Clearly define the problem you want to solve and identify the relevant data sets that can help you gain insights into the problem.
-
Consider the nature of the problem and choose the most appropriate analytical approach, such as forecasting, simulation, rules-based systems, or optimization.
-
Identify the predictive models that will help you forecast future outcomes and the prescriptive models that will help you optimize decisions.
-
Evaluate the trade-offs between predictive and prescriptive analytics. Predictive analytics helps you forecast future outcomes, while prescriptive analytics helps you optimize decisions. The optimal mix will depend on the problem you are trying to solve.
-
Develop a roadmap for the implementation. This will include deploying predictive and prescriptive analytics models and integrating data from multiple sources.
Step 2: Leverage Data Scientists
The next step is to hire data scientists or use those already available within the organization to develop predictive and prescriptive analytics models. The steps to hire data scientists or use those already available within the organization to develop predictive and prescriptive analytics models are as follows:
-
Determine the skills and expertise needed to develop predictive and prescriptive models, such as statistical analysis, machine learning, programming, and domain-specific knowledge.
-
Assess the skills and expertise of the existing workforce to determine if there are individuals who can be trained to develop predictive and prescriptive models.
- Create job descriptions and qualifications for new hires based on the required skills and expertise and source candidates from job boards, social media, professional networks, and referrals.
-
Conduct interviews and assessments and onboard them to the organization.
-
Provide training and development opportunities to new hires and existing employees to develop their skills and expertise in predictive and prescriptive analytics.
Alternatively, organizations can provide citizen data scientists with augmented analytics techniques to develop predictive and prescriptive models. This involves using analytics tools that enable business users to perform advanced analytics without needing specialized skills.
Suppose the organization does not have the necessary skills or resources to develop predictive and prescriptive models in-house. In that case, it may be necessary to consider using external data and analytics service providers. These providers can help fill gaps in skills or resources and jump-start learning and model building.
Step 3: Identify Complementary Skills
It is essential to identify complementary skills for rule-based systems and optimization techniques to fully leverage predictive and prescriptive analytics. Identifying complementary skills for rule-based systems and optimization techniques involves the following steps:
-
Assessing current skills of the organization's data analytics team. This includes identifying their strengths and weaknesses and any skills gaps that may exist in the organization.
-
Identifying required skills for rule-based systems and optimization techniques. This involves analyzing the organization's specific needs and determining the skills necessary to effectively utilize these techniques.
-
If there are skill gaps within the organization, the organization can hire new employees with the necessary skills or train existing employees.
-
Alternatively, the organization can partner with external service providers with advanced analytics expertise.
-
To ensure the most effective use of predictive and prescriptive analytics, building a diverse team with a range of skills and expertise is important. This can include data scientists, analysts, IT professionals, and business leaders.
By identifying complementary skills for rule-based systems and optimization techniques, organizations can ensure they have the expertise to fully leverage predictive and prescriptive analytics to drive business results.
Step 4: Develop a Process to Manage and Share Analytic Artifacts
More than 70% of CX leaders in a business find it difficult to develop programs that emphasize CX.
Developing a process to manage and share analytic artifacts is important to ensure that the organization can effectively leverage predictive and prescriptive analytics. Here are the steps to develop a process to manage and share analytic artifacts:
-
Establish a team responsible for managing and sharing analytic artifacts. This team should include data scientists, IT professionals, and business stakeholders.
-
Identify the analytic artifacts that need to be managed and shared, such as models, data sets, and documentation.
-
Develop a centralized repository for storing the artifacts. This can be a shared network drive or a cloud-based platform.
-
Implement a version control system to manage changes to the artifacts. This ensures that everyone works with the most up-to-date version and that changes can be tracked and reversed.
-
Develop a process for testing artifacts before they are deployed. This ensures that the artifacts are accurate and reliable.
-
Define a process for deploying the artifacts. This ensures that the artifacts are deployed in a consistent and controlled manner.
-
Implement access controls to ensure only authorized users can access the artifacts.
-
Develop documentation standards for the artifacts. This ensures that the artifacts are properly documented and that everyone can understand how to use them.
-
Provide training to users on how to access and use the artifacts. This ensures that everyone is able to use the artifacts effectively.
-
Monitor usage of the artifacts to ensure that they are being used effectively and that any issues are identified and addressed.
This may involve developing a centralized repository for analytic artifacts, such as models, data sets, and documentation, and implementing a version control, testing, and deployment process.
Step 5: Incorporate Packaged Analytic Applications
Another key step is incorporating packaged analytic applications that provide solutions specific to an industry, function, or analytics application. Here are the steps to incorporate packaged analytic applications:
-
Before selecting a packaged analytic application, it is important to identify the organization's specific needs. This may include determining the industry, function, or analytics application for which the application will be used.
-
Conduct research to identify available packaged analytic applications that meet the organization's needs. This may include reviewing industry publications, attending conferences, and engaging with vendors.
-
Once a list of potential applications has been identified, evaluate each application based on its functionality, ease of use, and integration with existing systems.
-
After evaluating the applications, select the most appropriate one for the organization.
-
Once the application has been selected, implement it by integrating it into the existing technology infrastructure.
-
Provide training to employees who will be using the application to ensure they are familiar with its functionality and can use it effectively.
-
Monitor the performance of the application and refine it as necessary to ensure it continues to meet the organization's needs.
These applications can help organizations accelerate the development and deployment of predictive and prescriptive models and provide a framework for ongoing model development and refinement.
Step 6: Partner with Service Providers With Advanced Analytics Expertise
Finally, organizations should consider partnering with service providers with specific advanced analytics expertise to help develop and deploy predictive and prescriptive models.
Here are some steps to consider when partnering with service providers with advanced analytics expertise:
-
Conducting thorough research on potential service providers and evaluating their expertise, track record, and customer satisfaction is essential.
-
Clearly define the project requirements, including the business problem to be solved, data sources, and desired outcomes. This will help potential service providers understand the project scope and develop a product proposal that aligns with your goals.
-
Request proposals from potential service providers based on your research and project requirements. Evaluate the proposals based on their technical approach, pricing, and ability to meet project deadlines.
-
Before finalizing a partnership, evaluate the provider's ability to work collaboratively with your organization and integrate with existing systems and processes. This can involve conducting interviews, requesting references, and evaluating past projects.
-
Once you have selected a service provider, develop a clear project plan outlining the scope, timeline, milestones, and deliverables. Both parties should review and agree upon this plan before beginning the project.
-
Establish clear communication channels and protocols to ensure that all project stakeholders are informed and involved throughout the project.
-
Regularly monitor the service provider's progress and performance to ensure that project milestones are met and that the project delivers the desired outcomes.
-
After the project's completion, conduct a review and refine the project's outcomes, including the:
-
effectiveness of the partnership
-
The accuracy of the models developed.
-
The overall impact on business operations.
This feedback can inform future partnerships and improve the organization's analytics capabilities.
These providers can bring deep expertise in specific industries or analytics applications and provide various services, including data preparation, model development, and model deployment.
Conclusion
Fusing predictive and prescriptive analytics is a powerful approach for gaining a comprehensive view of the customer journey by integrating various CX data sources and analyzing them to gain insights into customer behavior and preferences.
By leveraging both predictive and prescriptive analytics, organizations can create targeted marketing campaigns, enhance product design, and improve customer service, ultimately improving customer satisfaction and loyalty.
Implementing CX Analytics Fusion involves several key steps, including selecting the optimal mix of analytics capabilities, leveraging data scientists, identifying complementary skills, developing a process to manage and share analytic artifacts, incorporating packaged analytic applications, and partnering with service providers with advanced analytics expertise.
By following these steps, organizations can effectively leverage predictive and prescriptive analytics to drive business outcomes and gain a competitive advantage in today's data-driven business environment.
Read More: How Do Leading Management Consulting Firms Win New Businesses Using CX Analytics